(Miguel Candela / SOPA Images/Sip via Reuters Connect)
When describing the behavior of robotic systems, we tend to rely on anthropomorphisms. Cameras “see,” decision algorithms “think,” and classification systems “recognize.” But the use of such terms can set us up for failure, since they create expectations and assumptions that often do not hold, especially in the minds of people who have no training in the underlying technologies involved. This is particularly problematic because many of the tasks we envision for robotic technologies are typically ones that humans currently do (or could do) some part of. The natural tendency is to describe these tasks as a human would using the “skills” a human has—which may be very different from how a robot performs the task. If the task specification relies only on “human” specifications—without making clear the differences between “robotic” skills and “human” ones—then the chance of a misalignment between the human-based description of the task and what the robot actually does will increase.
Designing, procuring, and evaluating AI and robotic systems that are safe, effective, and behave in predictable ways represents a central challenge in contemporary artificial intelligence, and using a systematic approach in choosing the language that describes these systems is the first step toward mitigating risks associated with unexamined assumptions about AI and robotic capabilities. Specifically, actions we consider simple need to be broken down and their components carefully mapped to their algorithmic and sensor counterparts, while avoiding the pitfalls of anthropomorphic language. This serves two purposes. First, it helps to reveal underlying assumptions and biases by more clearly defining functionality. Second, it helps non-technical experts better understand the limitations and capabilities of the underlying technology, so they can better judge if it meets their application needs.
Ask a robot to pick up an apple
Consider, for example, a domain expert who has been tasked with finding a robotic or AI solution to a domain-specific problem of picking up apples from a table and putting them in a basket. The domain expert uses human-specific task terms (“see,” “pick,” “place”) to define what the robot should do (the procurement process). The domain expert might also provide testing and evaluation specifications—the robot should pick up at least 80% of the apples without bruising them.
Incorrect language use at this point can lead to a substantial misalignment between the desired specifications and what is actually implemented, since the understandings of the terms might differ between the procurer and the developer. “See an apple” gets translated to “identify red pixels,” “pick up” plus “don’t bruise” implies “don’t drop” to the domain expert, but not to the developer. This misalignment can be further exacerbated when the system is passed off to the operators implementing low-level parts of the robotic system. They might have yet another understanding of how the robot should work, based on the human-task description. Because the underlying implementation does not align with the (human-level) description of the system, the operators are unable to accurately predict the system behavior. This can lead to catastrophic failures, such as the operator assuming the system can do something it can’t, or the system doing something unexpected, such as assuming an image of a hot air balloon on a t-shirt is an apple.
Unfortunately, these anthropomorphisms are appealing, because they provide a common language between people with different specialties. We could avoid problems if the procurement specification pinned down the technical details: “a pixel value of (232, 3, 3),” but this is not possible without the procurement agent having detailed technical knowledge of the system. It’s easier to say “red,” since everyone knows that this is. However, at some point, it does have to be pinned down in order to implement it. In this implementation process, the resulting system may end up drifting substantially from the intent of the anthropomorphic term. This gets worse as the concepts get more subtle. While “red” is a slippery concept, “detect a person” is orders of magnitude worse.
We can illustrate this failure with a simple example. Let’s say the program manager requests a robot system that can see an apple and pick it up. The actual implementation is a camera that detects red pixels that form a rough circle. The robot uses two consecutive images to estimate the location of the apple, executes a path that moves the gripper to the apple, then closes the gripper fingers and lifts. When deployed, the robot mistakes the picture of a hot air balloon on a shirt and tries to drive the gripper through the person in an attempt to pick it up. This failure is not at all a surprise given the implementation description but would come as a shock to the person who was only told that the robot could “see apples and pick them up.” Many of the failures that seem to plague robots and AI systems are perfectly clear when described in terms of implementation details but seem inconceivably stupid when described using anthropomorphic language.
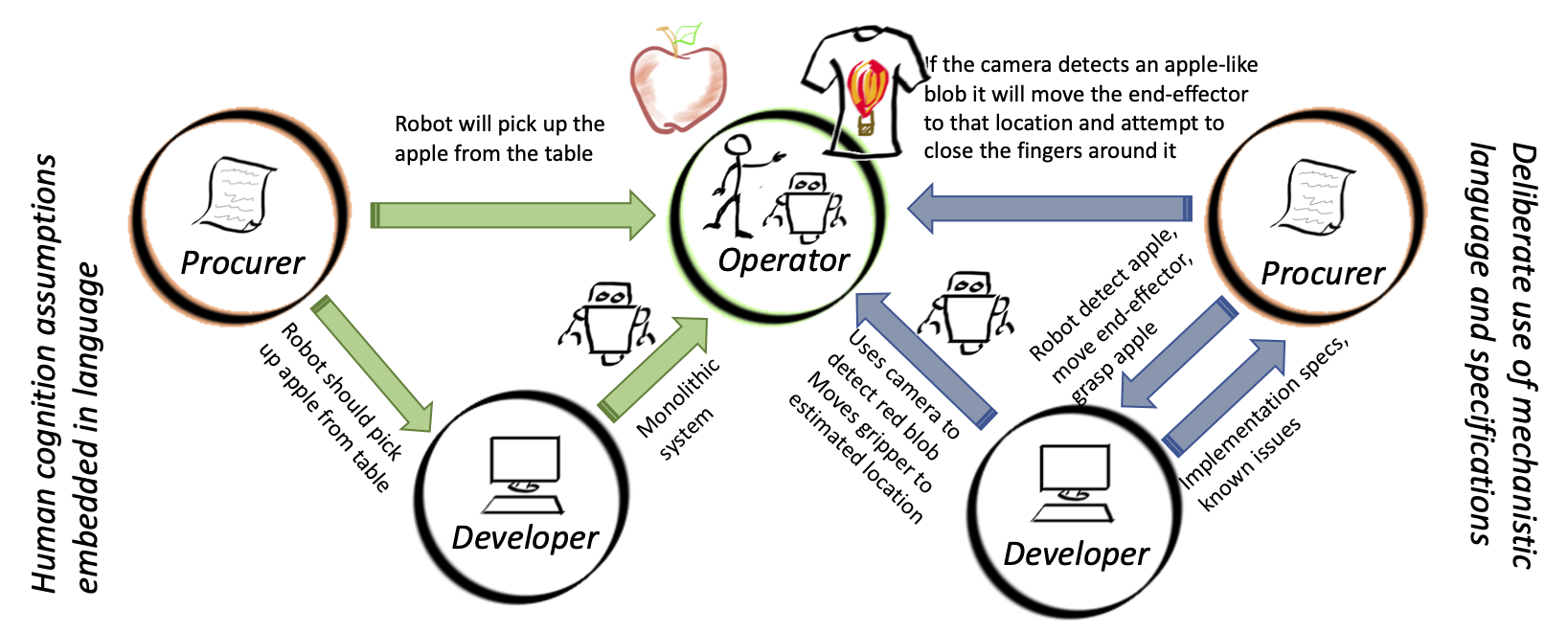
Dismantling the black box
Clear, non-anthropomorphic language is central to dismantling misconceptions of AI as a black box. The average AI or robotic system is still far less complex than the average bacterium, so why does the average person have difficulty reasoning about what these systems can (and cannot) do? This difficulty arises primarily because of language—specifically, the use of language that carries with it human-centric expectations and assumptions.
One could argue that the two statements “The robot sees an apple” and “The robot detects an object that has the appearance of an apple” are pretty much the same, but in their assumptions of cognitive ability, they are very different. “See” carries with it a host of internal models and assumptions: Apples are red or green, fit in the hand, smell like apples, crunch when you bite them, are found on trees and fruit bowls, etc. We are used to seeing apples in a wide variety of lighting conditions and varying view points—and we have some notion of the context in which they are likely to appear. We can separate out pictures of apples from paintings or cartoons. We can recognize other objects in a scene that tell us if something is likely to be an apple or another red object. In other words, we bring an entire internal representation of what an apple is when looking at an image—we don’t just see the pixels. “Detect,” on the other hand, connotes fewer internal assumptions and evokes, instead, the image of someone pointing a sensor at an apple and having it go “ding.” This is more akin to how a robot “sees” and how it internally represents an apple. A sensor (the camera) is pointed at the apple, and the numeric distribution of pixel values is examined. If the pixel values “match” (numerically) the previously-learned examples of pixel distributions for images labeled as “apples,” the algorithm returns the symbol “apple.” How does the algorithm get this set of example pixel distributions? Not by running around and picking up objects and seeing if they smell and taste like apples, but from millions of labeled images (thank you, Flickr). These images are largely taken with good lighting and from standard viewpoints, which means that the algorithm struggles to detect an apple in bad lighting and from odd angles, nor does it know how to distinguish between an image that matches its criteria of being an apple but isn’t one. Hence, it is more accurate to say that the robot has detected an object that has the appearance of an apple.
Phrasing the problem of picking up an apple using words such as “detect,” “move,” and “grasp” immediately reveals key questions in need of answers: What hardware is being used to do the detection? What are its known failures? What algorithms are being used? Instead, these problems can be addressed in a two-pronged approach: 1) Provide detailed specifications that clearly delineate (in lay terms) the underlying computer representations along with known failure cases and assumptions. 2) Select language terms that are mechanistic, not human-centric, and be very pedantic in describing system functionality. Explicitly defining nouns, adjectives, and verbs using their digital representation would greatly reduce the tendency of operators to assume that the software has their “human” internal representation of those terms. The spec sheet summaries of known failure cases would also improve the operator’s ability to reason about when and why the system might fail in its task. Using pedantic, mechanistic language can reinforce that these systems are not using (implied) human skill sets to do their tasks. On the procurement side—and working with developers to design testing and evaluation procedures—these detailed specifications plus pedantic language can help to uncover hidden assumptions and identify where (and how much) specific testing is required. This can be at the data set/sensor level (e.g., have you tested for low-light conditions?) or at a higher level (e.g., how do you know that the robot successfully picked up the apple?)
Critics might call this a “simple” example, and wonder where the AI is. In reality, this is one of the hardest things for a robot system to do (it’s just easy for us), and AI is embedded throughout—from the machine learning software that detects the apple to the path planning that gets the gripper to the apple. There is no “black box” AI that will just “do” what you want. Rather, every complex system is built from smaller components that can each be broadly characterized, tested, and evaluated. Correct use of mechanistic, pedantic language is a powerful tool to reveal those components and correctly bracket their capabilities.
Cindy M. Grimm is a professor in the School of Mechanical, Industrial, and Manufacturing Engineering at Oregon State University.